Infrared sensors-a commonly used imaging device. Unmanned aircraft vehicles-the most promising moving platform, each of them plays a vital role in their own field respectively. Surprisingly, the two devices are seldom combined in automatic ground vehicle detection task. Therefore ground vehicle detection in aerial imagery which aims at to seek the vehicles of aerial imagery has aroused wide academic concern and significant industrial application. However, due to the aerial imagery's low-resolution and fuzzy and ground vehicle detection's complexity, how to extract remarkable feature from them and handle pose variations, view changes as well as surrounding radiations remains a challenge. In fact, these typical abstract features extracted by convolutional neural network are more recognizable than engineering feature and these involved complex conditions can be learned and memorized before.
In this paper, we present a novel approach toward ground vehicle detection in aerial infrared images based on convolutional neural network instead of background segmentation or foreground extraction. Ground vehicle detection in aerial infrared imagery is firstly introduced by the UAV and the infrared sensor. Then a novel aerial moving platform is built to collect a large amount of aerial infrared images. It is mainly composed of the DJI MATRICE-100 and the FLIR TAU2 infrared sensor. Thus an aerial infrared vehicle database is unprecedentedly constructed, and we release this dataset on public website at https://shanxiliuxiaofei.github.io/. These samples record some common challenges that ground vehicle detection faces in practice, and can be used for the following researches in this field. Next, we build an end to end convolutional neural network, which can extract some typical abstract features. As these large amounts of recognized features be iteratively learned, a real-time ground vehicle mode is eventually constructed. It has the unique ability to detect both stationary vehicles and moving vehicles in real urban environments. We evaluate the proposed algorithm on some low-resolution aerial infrared images taken in real time. Experiments on the NPU_CS_UAV_IR_DATA database, which includes four different scenes, demonstrates that our method is effectiveness and efficiency to recognize the ground vehicles. And it can real-time accomplishes the task while achieves superior performances in leak and false alarm ratio.
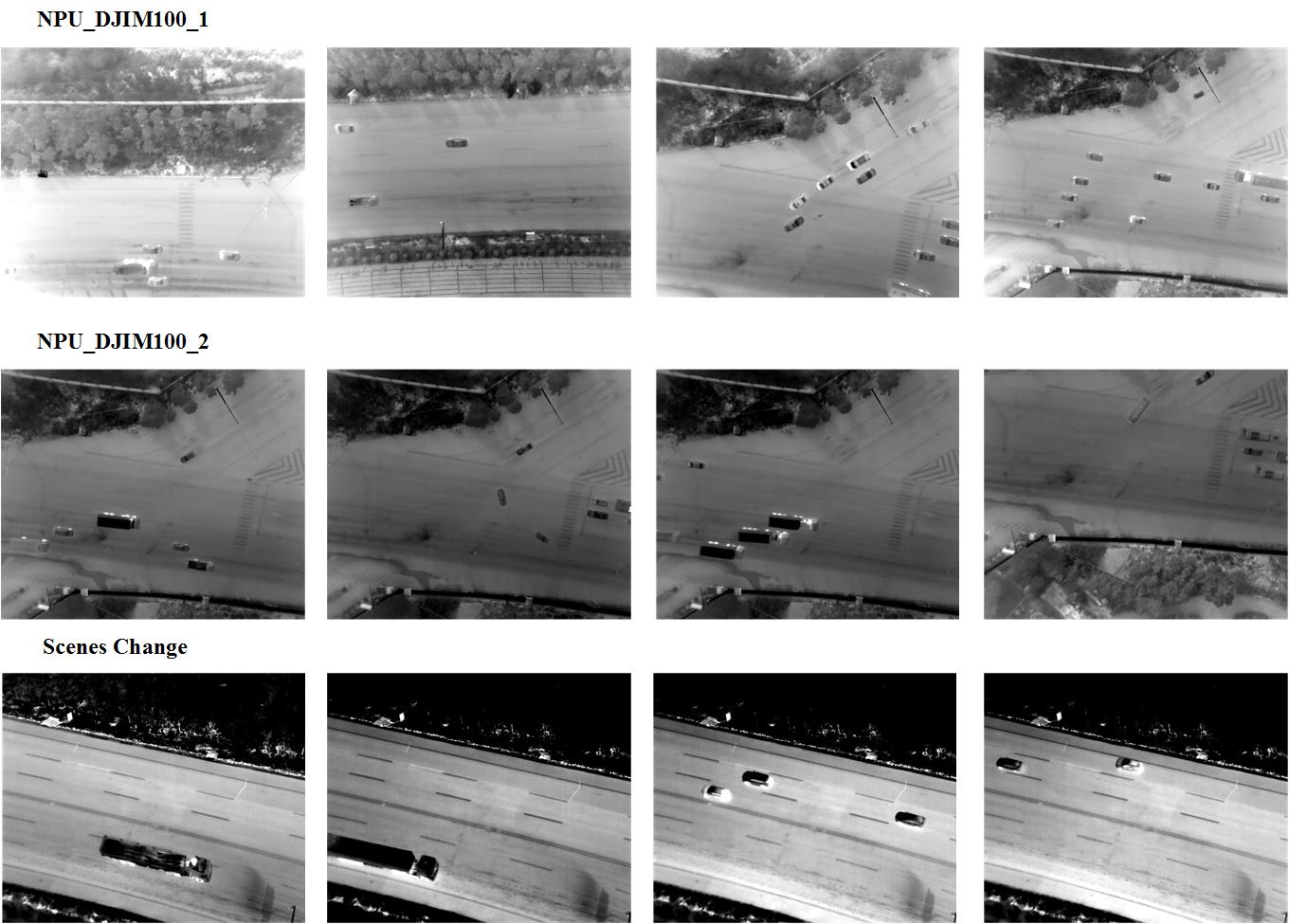
Dataset can be downloaded Here, which was caputred about 10 fps.The data is composed of Train Samples and Test Images. The Train Samples are divided into two parts(data1, data2). The Test Images contains NPU_DJM100_1, NPU_DJM100_2 and Scenes Change.
A simple demo video for Real-time Ground Vehicle Detection in Aerial Imagery Based on Convolutional Neural Network can be downloaded Here.
[1] Xiaofei Liu, Tao Yang and Jing Li. Real-time Ground Vehicle Detection in Aerial Imagery Based on Convolutional Neural Network, submitted to Electronics (under review) .